Beware of the Dangers of Dataschmozz in Machine Learning
Written on
Chapter 1: Understanding Dataschmozz
In the realm of professional wrestling, a "schmozz" refers to a chaotic match conclusion where numerous wrestlers invade the ring, resulting in confusion and a no-contest ruling. This type of ending is often seen as a lazy resolution, reflecting a promoter's inability to determine a clear victor or a failure to agree upon a proper conclusion. Schmozzes fail to advance wrestling narratives and are generally viewed as a waste of time, posing risks to already injury-prone wrestlers.
In the context of data analytics, I propose a term that encapsulates a similarly ineffective approach: the "dataschmozz." This term describes a careless and counterproductive method of addressing business challenges through machine learning, a trend that is unfortunately gaining traction due to misconceptions among business leaders regarding the nature of machine learning.
How Do Dataschmozzes Occur?
A dataschmozz often arises from a simplistic and naïve grasp of machine learning's capabilities, particularly among business leaders. The flawed reasoning typically includes the following assumptions:
- We have a business challenge to tackle.
- We possess abundant data pertinent to this challenge.
- By simply inputting this data into a machine learning model, we can derive insights to resolve the issue.
What's critical to recognize is that this approach neglects essential steps such as defining the problem, formulating hypotheses, and understanding the data's meaning and context. The result is an indiscriminate feeding of data into machine learning algorithms—a classic dataschmozz. The issue becomes exacerbated when data scientists or analysts fail to challenge this mindset, thereby hindering a more thoughtful and structured analysis.
Why Are Dataschmozzes Problematic?
Much like their wrestling counterparts, dataschmozzes represent a lazy and ineffective strategy for leveraging analytics. They often waste valuable time for data professionals and fail to enhance our comprehension of the issues at hand, frequently reaffirming already known facts. More alarmingly, these practices can lead to misleading conclusions that do not address the actual problem.
Strategic business challenges are frequently vague and poorly articulated from the start; applying models without properly defining them is akin to using a hacksaw for a haircut—seemingly quicker but ultimately disastrous! For instance, consider a scenario where we seek to understand the factors influencing job offers. The recruitment process likely involves multiple stages with varying inputs and decision-making criteria at each phase. Failing to account for this complexity and indiscriminately aggregating all available data may yield superficial insights, such as "candidates who reach the final stage are most likely to receive a job offer." This is not only unhelpful but also a clear indication that machine learning cannot replace critical thinking.
The effort involved in a dataschmozz can also be substantial. Business leaders often desire to incorporate numerous data sources, which may be challenging to access or stored in inconvenient formats. Data extraction might necessitate complex scripts or even manual effort, and merging disparate datasets while ensuring compliance with privacy standards adds another layer of complexity. Once this groundwork is laid, the data scientist, without clear direction beyond "try machine learning," may test a myriad of algorithms, hoping for an extraordinary discovery. If results are met with skepticism from business leaders due to misalignment with their preconceived notions, they may demand reanalysis on data subsets, prolonging the process and potentially consuming months of work.
Perhaps most concerning is that dataschmozzes can produce seemingly insightful outputs that are merely artifacts of the input data. For instance, if a company's recruitment strategy favors graduates and yields a high job offer rate, a poorly structured model might erroneously conclude that "having a college degree predicts job offers." Such findings can be dangerously misleading if misinterpreted.
For those familiar with the pitfalls of dataschmozzes, you may recognize elements of your experiences in this discussion. Newcomers to data science should remain vigilant for signs of potential dataschmozzes. It's crucial to advocate for clear problem definitions and structured analyses to prevent the inefficiencies and ineffectiveness that often accompany these scenarios. If this resonates with you or if you have additional thoughts to share, feel free to comment below!
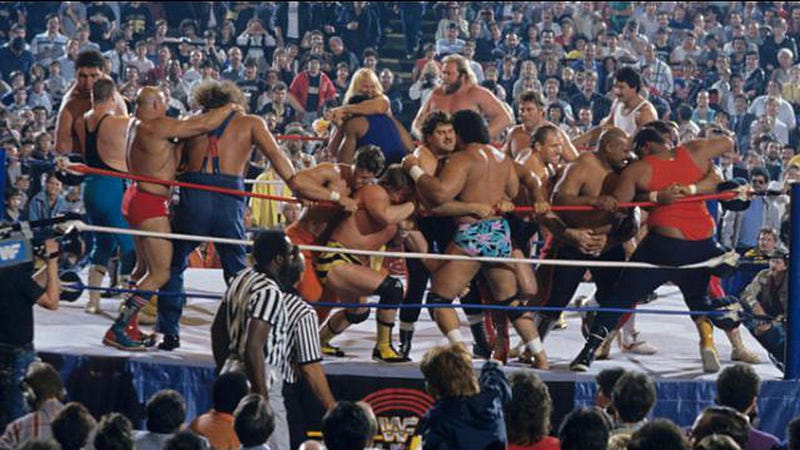
Chapter 2: The Consequences of Dataschmozz
This video titled "Loathsome Lies of Machine Learning" discusses the misconceptions surrounding machine learning and the dangers of misapplying it in business contexts.
In the video "Calling Bullshit 5.5: Criminal Machine Learning," the pitfalls of misusing machine learning in decision-making processes are examined, highlighting the risks associated with poorly structured analyses.