Decoding Depression: The Silent Signals Our Faces Convey
Written on
Chapter 1: Understanding Facial Expressions and Depression
Depression has often been a hidden struggle, concealed behind forced smiles and unspoken sorrow. Conventional diagnostic techniques, which depend heavily on personal accounts and clinical evaluations, frequently overlook the subtle cues of this mental health issue. However, what if our facial expressions could narrate our emotional state? Recent investigations are examining facial expressions as objective indicators of depression. By scrutinizing action units (AUs)—the minute movements of facial muscles—we can uncover patterns that reflect emotional conditions. This research focuses on the timing and dynamics of these expressions, comparing those of individuals experiencing depression with those of healthy individuals. The results indicate that certain AUs linked to sadness and happiness can provide significant insights into a person's mental well-being, paving the way for more precise and timely diagnoses.
Section 1.1: The Science Behind Facial Expressions
Facial Action Units (AUs) are fundamental components of our facial expressions. Each AU corresponds to a distinct muscle movement, such as raising an eyebrow or curling a lip. This research specifically examined AUs that are typically associated with emotions like sadness and happiness. For instance, AU1 (inner brow raiser) and AU15 (lip corner depressor) are frequently observed in expressions of sadness. By analyzing video recordings of participants, researchers were able to quantify the intensity and occurrence of these AUs. The findings revealed that those suffering from depression exhibited heightened AU1 and AU15 activity while showing reduced AU12 (lip corner puller), which is linked to happiness. This method provides a non-invasive and measurable way to detect depression.
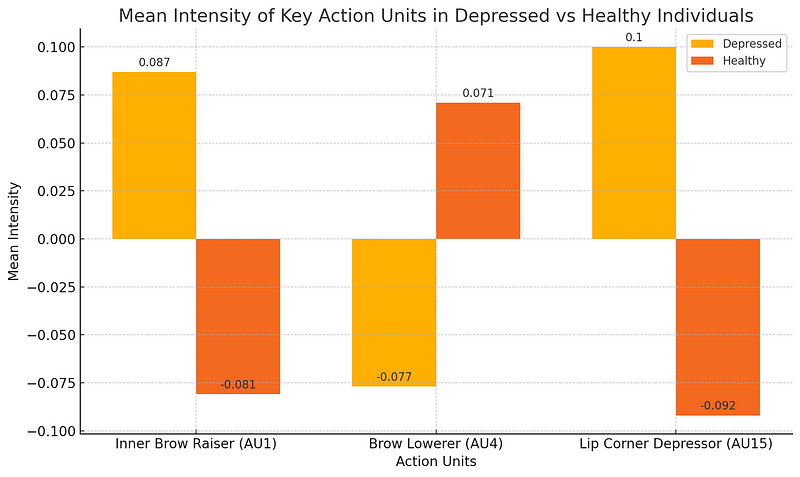
Section 1.2: Machine Learning Enhancements in Diagnosis
The incorporation of machine learning into facial expression analysis marks a remarkable leap in mental health diagnostics. Researchers employed various machine learning algorithms on the AU data, including Principal Component Analysis (PCA) and clustering techniques. These models successfully differentiated between depressed and non-depressed individuals by identifying patterns in facial expressions over time. The application of time-series classification models, such as Long Short-Term Memory (LSTM) networks, further improved predictive accuracy. This technological advancement not only provides objective insights but also has the potential to create automated screening tools, facilitating early intervention.
Chapter 2: The Significance of Facial Biomarkers
The ramifications of this research are substantial. By leveraging facial expressions as biomarkers, we can transcend subjective evaluations, moving towards a more objective, data-centric approach to diagnosing depression. This methodology could transform mental health care, allowing for earlier and more accurate detection of depression, which can lead to prompt interventions and improved patient outcomes. Additionally, the non-invasive nature of facial analysis means it can be seamlessly integrated into routine health checks, enabling continuous mental health monitoring. This breakthrough offers hope for a new understanding and treatment of depression, benefiting countless individuals worldwide.
The first video, "Don't Suffer from Your Depression in Silence" by Nikki Webber Allen, emphasizes the importance of recognizing and addressing depression openly. It explores how societal pressures can lead individuals to hide their struggles, and advocates for open dialogue and understanding about mental health.
The second video, "Workshop 05 - John Erwin - Suicide... The Silent Killer," addresses the critical issue of suicide as a result of untreated depression. It highlights the need for awareness, prevention strategies, and support systems to help those in distress.
Section 2.1: Quantifiable Expressions of Sadness
Research has established that certain facial action units (AUs), such as AU1 (inner brow raiser) and AU15 (lip corner depressor), are significantly more pronounced in individuals with depression. This quantification of sadness can provide concrete data that aids in diagnosing depression, establishing a scientific foundation for what has traditionally been subjective observation.
Section 2.2: Happiness as a Diagnostic Indicator
Conversely, the AU related to happiness, AU12 (lip corner puller), is less evident in depressed individuals. This decrease in expressions of joy serves as an additional measurable sign of mental health, underscoring the subtle yet telling distinctions in how emotions manifest on our faces. It serves as a powerful reminder of the deep connections between our mental states and physical expressions.
Section 2.3: Advancements in Diagnostic Precision
Machine learning algorithms, including PCA and LSTM networks, can analyze variations in facial expressions over time, significantly enhancing the accuracy of depression diagnoses. These models can detect subtle changes in expressions that might escape human observation, making them invaluable tools in the field of mental health care. This technological innovation brings us closer to reliable and objective diagnostic methodologies.
Section 2.4: Non-Invasive Screening Opportunities
Utilizing facial expressions as biomarkers presents a non-invasive avenue for depression screening. Unlike traditional approaches that depend on self-reported questionnaires and clinical interviews, facial analysis can be performed without direct interaction, making it less intrusive and more comfortable for patients. This strategy could facilitate more frequent and routine mental health evaluations.
Section 2.5: The Promise of Real-Time Monitoring
The ability to assess facial expressions in real-time opens the door for continuous mental health monitoring. This could be particularly advantageous in environments like schools, workplaces, or even through smartphone applications, offering immediate feedback and early warning signals for depression. Such real-time evaluations could lead to swifter interventions and improved mental health outcomes.
The Future of Mental Health Care
The potential of utilizing facial expressions as biomarkers for depression represents a transformative shift in mental health care. This innovative strategy promises to enhance the accuracy and timeliness of diagnoses, ultimately leading to improved outcomes for those affected by depression. By harnessing technological advancements, we can turn our facial expressions into windows of insight, providing a clearer understanding of our mental states. This progress not only deepens our comprehension of depression but also inspires a future where mental health care is more accessible, compassionate, and effective. The quest for a world where every smile and frown can be interpreted in its true context is just beginning, holding the promise of a brighter, more emotionally aware future.
About Disruptive Concepts
Welcome to @Disruptive Concepts — your portal to the future of technology. Subscribe for new insightful videos every Saturday! Watch us on YouTube.